ibcbet
IBCBET: Agen Daftar IBCBET Judi Bola Resmi Terpercaya | Link IBCBET Login
IBCBET: Agen Daftar IBCBET Judi Bola Resmi Terpercaya | Link IBCBET Login
Couldn't load pickup availability

IBCBET: Agen Daftar IBCBET Judi Bola Resmi Terpercaya | Link IBCBET Login
IBCBET merupakan situs judi bola online resmi terpercaya dan terbesar di dunia yang sudah sangat populer sejak lama. Situs ibcbet taruhan bola juga menyediakan berbagai layanan bermain judi bola online paling lengkap. Bahkan untuk ketersediaan pasaran taruhan judi bola online yang disediakan oleh agen ibcbet Indonesia juga tergolong paling masuk akal. Hampir semua jenis permainan judi bola resmi selalu dihadirkan untuk penikmat taruhan bola uang asli. Tidak heran jika link ibcbet terbaru selalu menjadi pencarian utama di kalangan penggemar judi taruhan bola di Indonesia. Untuk daftar ibcbet juga tergolong mudah dan cepat, Bettor hanya perlu mencari situs judi bola resmi terpercaya agen ibcbet Indonesia saja untuk memiliki akun ibcbet login. Dimana pemain juga tidak perlu khawatir sulit untuk memasang taruhan judi bola online mengingat link alternatif ibcbet yang tidak diblokir selalu di informasikan kepada pemain. Inilah alasan mengapa situs ibcbet terpercaya di Indonesia masih menjadi salah satu bandar bola online terpercaya dan terbaik.
Taruhan bola ibcbet resmi juga selalu menyediakan berbagai layanan terbaik dan berkualitas kepada penikmat judi bola di Indonesia. Hal ini terlihat dari keunggulan situs ibcbet Indonesia yang menyediakan layanan 24 jam online. Bahkan untuk opsi deposit judi bola yang disediakan oleh bandar bola ibcbet juga tergolong lengkap. Bettor tidak hanya bisa main judi bola ibcbet menggunakan transfer bank saja, Melainkan juga ibcbet deposit pulsa 10 ribu tanpa potongan. Nah, Keuntungan hingga keunggulan dari agen resmi ibcbet Indonesia ini tentu sangat jarang bisa didapat oleh bettor bersama situs judi bola terpercaya di Indonesia yang lain. Ibcbet yang juga dikenal dengan nama maxbet Indonesia ini memang sudah sejak lama selalu memperhatikan kenyamanan bermain. Tidak heran jika agen maxbet juga selalu memberikan informasi link ibcbet terbaru kepada pemain.
Agen ibcbet Indonesia juga dikenal oleh penikmat taruhan bola sebagai salah satu situs judi bola online resmi dan terpercaya saat ini. Ya, Berbagai fasilitas bermain judi bola online resmi hingga proses daftar akun ibcbet yang mudah menjadi alasan utama. Disisi lain ketersediaan link ibcbet login untuk pemain juga menjadi layanan terbaik yang pernah dihadirkan oleh situs judi bola resmi di Indonesia. Seperti yang kita ketahui, Seringkali penggemar taruhan judi bola kesulitan untuk bermain karena masalah link alternatif ibcbet yang sulit di dapat. Nah, Menariknya melalui agen ibcbet resmi di Indonesia, Kini bettor bisa mendapatkan berbagai fasilitas terbaik ini. Bahkan saat ini agen resmi ibcbet terpercaya juga menawarkan bonus seperti cashback hingga rollingan kepada pemain. Tentunya keuntungan bermain judi bola ibcbet seperti sangat dibutuhkan oleh pemain untuk menutupi sedikit kerugian ketika taruhan bola online.
Situs judi bola resmi terpercaya seperti bandar bola ibcbet memang sampai saat ini masih menjadi pilihan terbaik karena berbagai alasan. Mulai dari kenyamanan bermain judi taruhan bola hingga kemudahan bettor mencari link judi bola untuk bermain. Situs ibcbet resmi di Indonesia bahkan sampai memberikan akses mudahnya daftar ibcbet mobile secara mudah dan cepat. Ya, Cukup menggunakan layanan live chat 24 jam yang disediakan oleh agen ibcbet Indonesia, Maka kalian sudah bisa mendapatkan akun ibcbet login. Tidak hanya itu saja, Link alternatif ibcbet mobile login juga bisa didapatkan secara gratis sehingga memudahkan bettor untuk memasang taruhan judi bola resmi. Melalui keunggulan demi keunggulan yang disediakan oleh agen ibcbet Indonesia, Tidak heran mereka menjadi salah satu daftar situs judi bola resmi dan terpercaya paling banyak peminat sampai saat ini.
Share
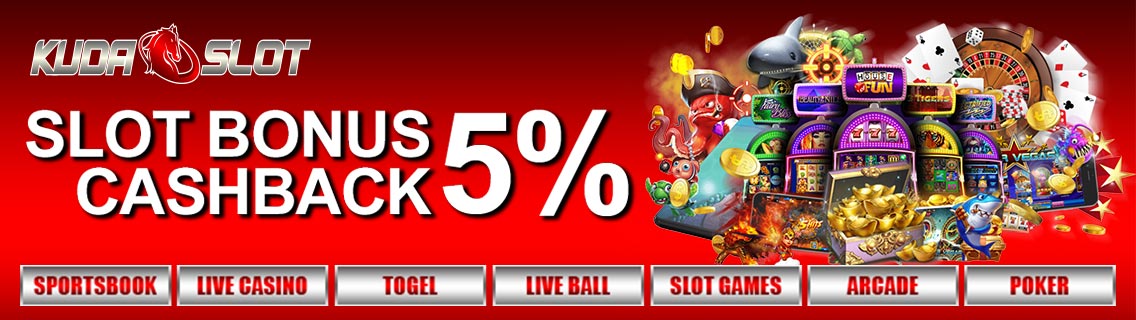